AI and Smart Customer Services: Revolutionizing the Customer Experience
Keywords:
Artificial Intelligence; Chatbots; Customer Service; Personalization; Predictive AnalyticsAbstract
The advent of artificial intelligence (AI) has significantly transformed customer service, enhancing efficiency, personalization, and customer satisfaction. This paper explores AI integration in customer service through technologies like chatbots, virtual assistants, predictive analytics, and sentiment analysis. These tools automate tasks, provide instant responses, and deliver tailored experiences, significantly boosting customer satisfaction and loyalty. However, challenges such as data privacy, job displacement, and AI biases pose significant concerns. Ensuring robust data protection, addressing potential job losses, and developing fair algorithms are crucial to overcoming these obstacles. This study provides a comprehensive overview of AI-driven smart customer services, highlighting the balance between leveraging AI for improved customer experiences and navigating associated ethical dilemmas. Future advancements in AI and natural language processing promise even more sophisticated and intuitive solutions, paving the way for a future where AI seamlessly integrates with human interactions to provide superior service.
References
Acquisti, A., Brandimarte, L., & Loewenstein, G. (2015). Privacy and human behavior in the age of information. Science, 347(6221), 509-514. https://doi.org/10.1126/science.aaa1465
Adamopoulou, E., Moussiades, L. (2020). An overview of chatbot technology. In Maglogiannis, I., Iliadis, L., Pimenidis, E. (eds) Artificial intelligence applications and innovations. AIAI 2020. IFIP Advances in Information and Communication Technology, vol 584. Springer, Cham. https://doi.org/10.1007/978-3-030-49186-4_31
Angwin, J., Larson, J., Mattu, S., & Kirchner, L. (2016). Machine bias. Retrieved May 19, 2024 from https://www.propublica.org/article/machine-bias-risk-assessments-in-criminal-sentencing
Atzori, L., Iera, A., & Morabito, G. (2017). The Internet of Things: A survey. Computer Networks, 54(15), 2787-2805. https://doi.org/10.1016/j.comnet.2010.05.010
Barocas, S., Hardt, M., & Narayanan, A. (2023). Fairness and machine learning: Limitations and opportunities. MIT Press.
Binns, R. (2018). Fairness in machine learning: Lessons from political philosophy. In Proceedings of the 2018 Conference on Fairness, Accountability, and Transparency (pp. 149-159). https://doi.org/10.1145/3287560.3287598
Brynjolfsson, E., & McAfee, A. (2014). The second machine age: Work, progress, and prosperity in a time of brilliant technologies. WW Norton & Company.
Buolamwini, J., & Gebru, T. (2018). Gender shades: Intersectional accuracy disparities in commercial gender classification. In Conference on Fairness, Accountability and Transparency (pp. 77-91). https://doi.org/10.1145/3287560.3287596
Caliskan, A., Bryson, J. J., & Narayanan, A. (2017). Semantics derived automatically from language corpora contain human-like biases. Science, 356(6334), 183-186. https://doi.org/10.1126/science.aal4230
Cavoukian, A. (2016). The 7 foundational principles of privacy by design. Information and Privacy Commissioner of Ontario, Canada. Retrieved May 19, 2024 from https://www.ipc.on.ca/wp-content/uploads/resources/7foundationalprinciples.pdf
Chen, Y., Chiang, R. H., & Storey, V. C. (2019). Business intelligence and analytics: From big data to big impact. MIS Quarterly, 36(4). https://doi.org/10.2307/41703503
Davenport, T., & Ronanki, R. (2018). Artificial intelligence for the real world. Harvard Business Review, 96(1), 108-116.
Devlin, J., Chang, M. W., Lee, K., & Toutanova, K. (2019). BERT: Pre-training of deep bidirectional transformers for language understanding. arXiv preprint arXiv:1810.04805.
Devlin, J., Chang, M. W., Lee, K., & Toutanova, K. (2019). BERT: Pre-training of deep bidirectional transformers for language understanding. NAACL-HLT. https://doi.org/10.18653/v1/N19-1423
Friedman, B., & Nissenbaum, H. (1996). Bias in computer systems. ACM Transactions on Information Systems (TOIS), 14(3), 330-347. https://doi.org/10.1145/230538.230561
Golla, B., & Kahn, J. (2019). Protecting privacy in a digital age: Report of the expert panel on privacy. Council of Canadian Academies. Retrieved May 19, 2024 from https://cca-reports.ca/reports/protecting-privacy-in-a-digital-age/
Gomez-Uribe, C. A., & Hunt, N. (2016). The Netflix recommender system: Algorithms, business value, and innovation. ACM Transactions on Management Information Systems (TMIS), 6(4), 1-19. https://doi.org/10.1145/2843948
Goodman, B., & Flaxman, S. (2017). European Union regulations on algorithmic decision-making and a "right to explanation". AI Magazine, 38(3), 50-57. https://doi.org/10.1609/aimag.v38i3.2741
Gretzel, U., Fesenmaier, D. R., Formica, S., & O'Leary, J. T. (2015). Searching for the future: Challenges faced by destination marketing organizations. Journal of Travel Research, 45(2), 116-126. https://doi.org/10.1177/0047287506291598
He, W., Zha, S., & Li, L. (2018). Social media competitive analysis and text mining: A case study in the pizza industry. International Journal of Information Management, 33(3), 464-472. https://doi.org/10.1016/j.ijinfomgt.2013.01.001
Holstein, K., Wortman Vaughan, J., Daumé III, H., Dudik, M., & Wallach, H. (2019). Improving fairness in machine learning systems: What do industry practitioners need? In Proceedings of the 2019 CHI Conference on Human Factors in Computing Systems (pp. 1-16). https://doi.org/10.1145/3290605.3300830
Hoy, M. B. (2018). Alexa, Siri, Cortana, and more: An introduction to voice assistants. Medical Reference Services Quarterly, 37(1), 81-88. https://doi.org/10.1080/02763869.2018.1404391
Hoy, M. B. (2018). Alexa, Siri, Cortana, and More: An introduction to voice assistants. Medical Reference Services Quarterly, 37(1), 81-88. https://doi.org/10.1080/02763869.2018.1404391
Huang, M. H., & Rust, R. T. (2018). Artificial intelligence in service. Journal of Service Research, 21(2), 155-172. https://doi.org/10.1177/10946705177524
Junqué de Fortuny, E., Martens, D., & Provost, F. (2013). Predictive modeling with big data: is bigger really better? Big Data, 1(4), 215-226. https://doi.org/10.1089/big.2013.003
Kharde, V., & Sonawane, S. (2016). Sentiment analysis of twitter data: A survey of techniques. International Journal of Computer Applications, 139(11), 5-15. https://doi.org/10.48550/arXiv.1601.06971
Kietzmann, J., Paschen, J., & Treen, E. R. (2018). Artificial intelligence in advertising. Journal of Advertising Research, 58(3), 263-267. https://doi.org/10.2501/JAR-2018-035
Li, S., Xu, L. D., & Zhao, S. (2018). The Internet of Things: A survey. Information Systems Frontiers, 17(2), 243-259. https://doi.org/10.1007/s10796-014-9492-7
Liu, B. (2015). Sentiment analysis: Mining opinions, sentiments, and emotions. Cambridge University Press. https://doi.org/10.1017/CBO9781139084789
Liu, B., Wang, Y., & Liu, W. (2019). Towards explainable AI: A survey on interpreting the outputs of AI models. arXiv preprint arXiv:1907.13545. https://doi.org/10.48550/arXiv.1907.13545
Madras, D., Creager, E., Pitassi, T., & Zemel, R. (2018). Learning adversarially fair and transferable representations. In Proceedings of the 35th International Conference on Machine Learning (pp. 3381-3390). https://doi.org/10.48550/arXiv.1802.06309
Manyika, J., et al. (2017). Jobs lost, jobs gained: Workforce transitions in a time of automation. McKinsey Global Institute.
Manyika, J., Lund, S., Chui, M., Bughin, J., Woetzel, J., Batra, P., ... & Sanghvi, S. (2017). Jobs lost, jobs gained: Workforce transitions in a time of automation. McKinsey Global Institute, 150(1), 1-148.
Manyika, J., Lund, S., Chui, M., Bughin, J., Woetzel, J., Batra, P., ... & Sanghvi, S. (2017). Jobs lost, jobs gained: Workforce transitions in a time of automation. McKinsey Global Institute, 150(1), 1-148.
Mehrabi, N., Morstatter, F., Saxena, N., Lerman, K., & Galstyan, A. (2021). A survey on bias and fairness in machine learning. ACM Computing Surveys (CSUR), 54(6), 1-35. https://doi.org/10.1145/3457607
Paschen, J., Kietzmann, J., & Kietzmann, T. C. (2019). Artificial intelligence (AI) and its implications for market knowledge in B2B marketing. Journal of Business & Industrial Marketing, 34(7), 1410-1419. https://doi.org/10.1108/JBIM-10-2018-0295
Picard, R. W. (2017). Affective computing. MIT Press.
Porter, M. E., & Heppelmann, J. E. (2015). How smart, connected products are transforming companies. Harvard Business Review, 93(10), 96-114.
Ribeiro, M. T., Singh, S., & Guestrin, C. (2016). "Why should I trust you?" Explaining the predictions of any classifier. In Proceedings of the 22nd ACM SIGKDD International Conference on Knowledge Discovery and Data Mining (pp. 1135-1144). https://doi.org/10.1145/2939672.2939778
Shin, D. (2021). The effects of explainability and causability on perception, trust, and acceptance: Implications for explainable AI. International Journal of Human-Computer Studies, 146, 102551. https://doi.org/10.1016/j.ijhcs.2020.102551
Smith, B., & Linden, G. (2017). Two decades of recommender systems at Amazon.com. IEEE Internet Computing, 21(3), 12-18. https://doi.org/10.1109/MIC.2017.72
Solove, D. J. (2021). The myth of the privacy paradox. The George Washington Law Review, 89, 1.
Susskind, R. (2019). Online courts and the future of justice. Oxford University Press.
Wang, Y., Kung, L., & Byrd, T. A. (2018). Big data analytics: Understanding its capabilities and potential benefits for healthcare organizations. Technological Forecasting and Social Change, 126, 3-13. https://doi.org/10.1016/j.techfore.2015.12.019
West, D. M. (2018). The future of work: Robots, AI, and automation. Brookings Institution Press.
Wirtz, J., & Zeithaml, V. (2018). Artificial intelligence in service: Skepticism, satisfaction, and trust. Journal of Service Management, 29(1), 4-11. https://doi.org/10.1108/JOSM-04-2017-0094
Wirtz, J., & Zeithaml, V. (2018). Cost-effective service excellence. Journal of the Academy of Marketing Science, 46, 59-80. https://doi.org/10.1007/s11747-017-0560-7
Wu, Y., Schuster, M., Chen, Z., Le, Q. V., Norouzi, M., Macherey, W., ... & Dean, J. (2016). Google's neural machine translation system: Bridging the gap between human and machine translation. arXiv preprint arXiv:1609.08144. https://doi.org/10.48550/arXiv.1609.08144
Zhou, J., Li, L., Dong, L., Liu, Q., & Wei, F. (2020). Unified pre-training for natural language understanding and generation. arXiv preprint arXiv:2002.12804. https://doi.org/10.48550/arXiv.2002.12804
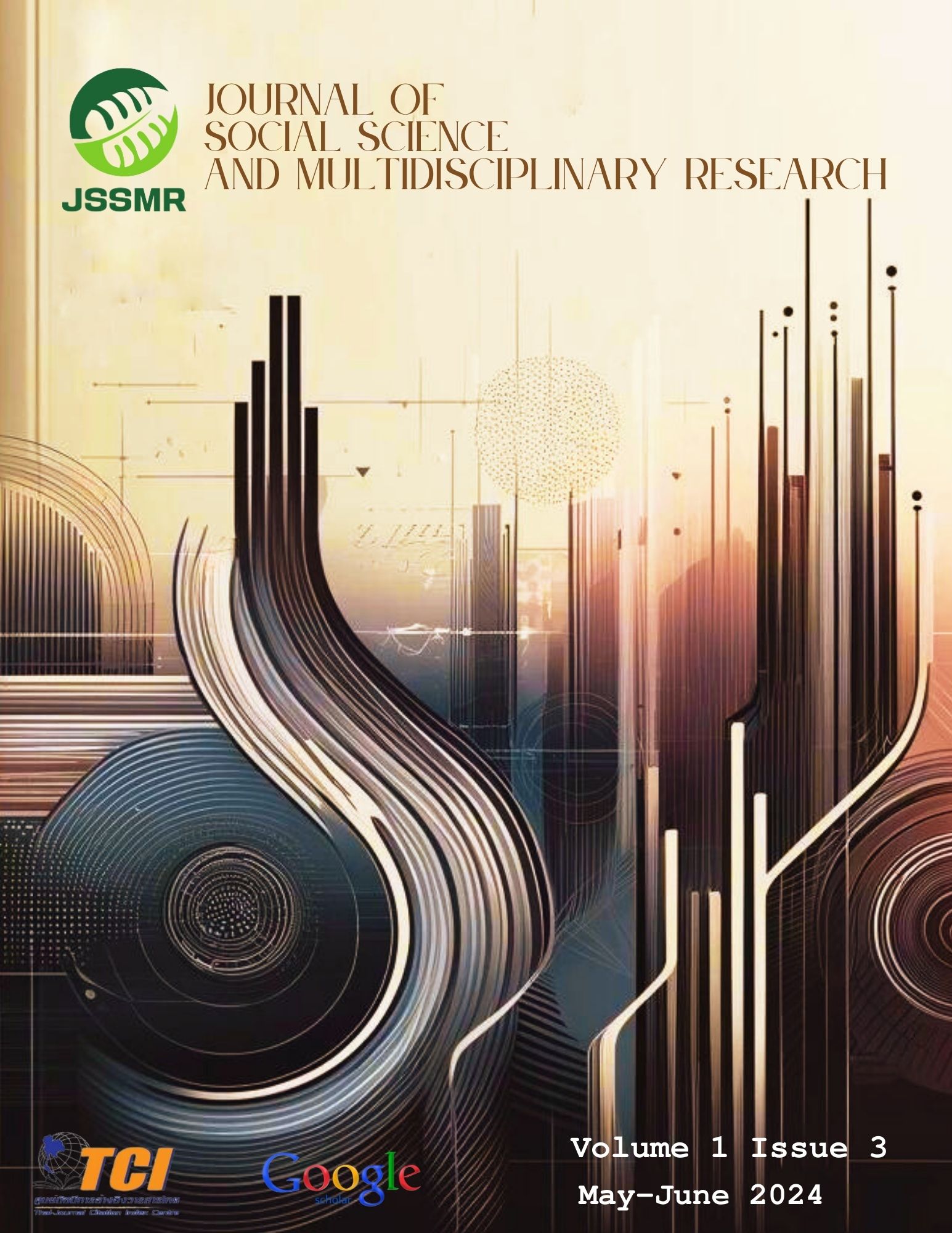